Hoy me gustar铆a hablar sobre c贸mo desempacar listas anidadas de profundidad indefinida. Esta es una tarea bastante no trivial, por lo que me gustar铆a contarles aqu铆 qu茅 son las implementaciones, sus ventajas y desventajas y una comparaci贸n de su rendimiento.
El art铆culo constar谩 de varias secciones a continuaci贸n:
- Las funciones
- Datos
- Resultados
- Conclusiones
Parte 1. Funciones
Implementaciones prestadas
outside_flatten_1
Implementaci贸ndef outer_flatten_1(array: Iterable) -> List: """ Based on C realization of this solution More on: https://iteration-utilities.readthedocs.io/en/latest/generated/deepflatten.html https://github.com/MSeifert04/iteration_utilities/blob/384948b4e82e41de47fa79fb73efc56c08549b01/src/deepflatten.c """ return deepflatten(array)
Tom茅 esta funci贸n para analizar desde un paquete externo, iteration_utilities.
La implementaci贸n se realiz贸 en C, dejando a Python una interfaz de llamada de funci贸n de alto nivel.
La implementaci贸n de la funci贸n en C es bastante engorrosa, puede verla haciendo clic en el enlace del spoiler. La funci贸n es un iterador.
>>> from typing import Iterator, Generator >>> from iteration_utilities import deepflatten >>> isinstance(deepflatten(a), Iterator) True >>> isinstance(deepflatten(a), Generator) False
Es dif铆cil decir sobre la complejidad del algoritmo implementado aqu铆, por lo que dejo este inter茅s a los usuarios de Habr.
En general, tambi茅n me gustar铆a se帽alar que todas las dem谩s funciones de esta biblioteca son bastante r谩pidas y tambi茅n se implementan en C.
exterior_flatten_2
Implementaci贸n def outer_flatten_2(array: Iterable) -> List: """ recursive algorithm, vaguely reminiscent of recursion_flatten. Based on next pattern: .. code:: python try: tree = iter(node) except TypeError: yield node more on: https://more-itertools.readthedocs.io/en/stable/api.html#more_itertools.collapse """ return collapse(array)
La implementaci贸n de este m茅todo de desempaquetar listas anidadas tambi茅n se encuentra en el paquete externo, es decir, more_itertools.
La funci贸n se realiza en Python puro, pero no es 贸ptima, en mi opini贸n. Se puede ver una implementaci贸n detallada en el enlace de documentaci贸n.
La complejidad de este algoritmo es O (n * m).
Implementaciones propias
niccolum_flatten
Implementaci贸n def niccolum_flatten(array: Iterable) -> List: """ Non recursive algorithm Based on pop/insert elements in current list """ new_array = array[:] ind = 0 while True: try: while isinstance(new_array[ind], list): item = new_array.pop(ind) for inner_item in reversed(item): new_array.insert(ind, inner_item) ind += 1 except IndexError: break return new_array
Cuando de alguna manera habl茅 sobre los telegramas al p煤blico @ru_python_beginners sobre la implementaci贸n de desempaquetar listas anidadas de anidamiento indefinido, propuse mi propia versi贸n.
Consiste en el hecho de que copiamos la lista original (para no cambiarla), y luego en el bucle while True verificamos mientras el elemento es una lista: lo revisamos e insertamos el resultado desde el principio.
S铆, ahora entiendo que no se ve 贸ptimo y dif铆cil, porque la reindexaci贸n ocurre cada vez (ya que agregamos y eliminamos de la parte superior de la lista), sin embargo, esta opci贸n tambi茅n tiene derecho a la vida y verificaremos su implementaci贸n junto con el resto.
La complejidad de este algoritmo es O (n ^ 3 * m) debido a la reconstrucci贸n de la lista dos veces por cada iteraci贸n aprobada
tishka_flatten
Implementaci贸n def tishka_flatten(data: Iterable) -> List: """ Non recursive algorithm Based on append/extend elements to new list """ nested = True while nested: new = [] nested = False for i in data: if isinstance(i, list): new.extend(i) nested = True else: new.append(i) data = new return data
Uno de los primeros tambi茅n mostr贸 la implementaci贸n de Tishka17 . Consiste en el hecho de que dentro del ciclo while anidado itera sobre la lista existente con la clave nested = False, y si al menos una vez tiene una hoja, deja la bandera anidada = True y extiende esta hoja a la lista. En consecuencia, resulta que para cada ejecuci贸n establece un nivel de anidamiento, cu谩ntos niveles de anidamiento habr谩, habr谩 tantos pases a trav茅s del ciclo. Como se puede ver en la descripci贸n, no es el algoritmo m谩s 贸ptimo, pero a煤n as铆, es diferente del resto.
La complejidad de este algoritmo es O (n * m).
zart_flatten
Implementaci贸n def zart_flatten(a: Iterable) -> List: """ Non recursive algorithm Based on pop from old and append elements to new list """ queue, out = [a], [] while queue: elem = queue.pop(-1) if isinstance(elem, list): queue.extend(elem) else: out.append(elem) return out[::-1]
Un algoritmo tambi茅n sugerido por uno de los participantes experimentados del chat. En mi opini贸n, es bastante simple y limpio. Su significado es que si el elemento es una lista, agregamos el resultado al final de la lista original, y si no, lo agregamos a la salida. Lo llamar茅 debajo del mecanismo extender / agregar. Como resultado, mostramos la lista plana resultante resultante invertida para preservar el orden original de los elementos.
La complejidad de este algoritmo es O (n * m).
recursive_flatten_iterator
Implementaci贸n def recursive_flatten_iterator(arr: Iterable) -> Iterator: """ Recursive algorithm based on iterator Usual solution to this problem """ for i in arr: if isinstance(i, list): yield from recursion_flatten(i) else: yield i
Probablemente la soluci贸n m谩s com煤n a este problema es a trav茅s de la recursividad y el rendimiento de. Poco se puede decir: el algoritmo parece bastante simple y eficiente, aparte del hecho de que se realiza a trav茅s de la recursi贸n y, con un gran anidamiento, puede haber una pila de llamadas bastante grande.
La complejidad de este algoritmo es O (n * m).
recursive_flatten_generator
Implementaci贸n def recursive_flatten_generator(array: Iterable) -> List: """ Recursive algorithm Looks like recursive_flatten_iterator, but with extend/append """ lst = [] for i in array: if isinstance(i, list): lst.extend(recursive_flatten_list(i)) else: lst.append(i) return lst
Esta funci贸n es bastante similar a la anterior, solo se ejecuta no a trav茅s de un iterador, sino a trav茅s de un mecanismo recursivo de extensi贸n / adici贸n.
La complejidad de este algoritmo es O (n * m).
tishka_flatten_with_stack
Implementaci贸n def tishka_flatten_with_stack(seq: Iterable) -> List: """ Non recursive algorithm Based on zart_flatten, but build on try/except pattern """ stack = [iter(seq)] new = [] while stack: i = stack.pop() try: while True: data = next(i) if isinstance(data, list): stack.append(i) i = iter(data) else: new.append(data) except StopIteration: pass return new
Un algoritmo m谩s que proporcion贸 Tishka, que, de hecho, es muy similar a zart_flatten, sin embargo, se implement贸 all铆 a trav茅s de un mecanismo simple de extensi贸n / adici贸n, luego a trav茅s de la iteraci贸n en un bucle infinito en el que se usa este mecanismo. Por lo tanto, result贸 algo similar a zart_flatten, pero a trav茅s de la iteraci贸n y mientras True
La complejidad de este algoritmo es O (n * m).
Parte 2. Datos
Para probar la efectividad de estos algoritmos, necesitamos crear funciones para la generaci贸n autom谩tica de listas de un determinado anidamiento, que he tratado con 茅xito y le mostraremos el resultado a continuaci贸n:
create_data_decreasing_depth
Implementaci贸n def create_data_decreasing_depth( data: Union[List, Iterator], length: int, max_depth: int, _current_depth: int = None, _result: List = None ) -> List: """ creates data in depth on decreasing. Examples: >>> data = create_data_decreasing_depth(list(range(1, 11)), length=5, max_depth=3) >>> assert data == [[[1, 2, 3, 4, 5], 6, 7, 8, 9, 10]] >>> data = create_data_decreasing_depth(list(range(1, 11)), length=2, max_depth=3) >>> assert data == [[[1, 2], 3, 4], 5, 6], [[7, 8,] 9, 10]] """ _result = _result or [] _current_depth = _current_depth or max_depth data = iter(data) if _current_depth - 1: _result.append(create_data_decreasing_depth( data=data, length=length, max_depth=max_depth, _current_depth=_current_depth - 1, _result=_result)) try: _current_length = length while _current_length: item = next(data) _result.append(item) _current_length -= 1 if max_depth == _current_depth: _result += create_data_decreasing_depth( data=data, length=length, max_depth=max_depth) return _result except StopIteration: return _result
Esta funci贸n crea listas anidadas con anidamiento decreciente.
>>> data = create_data_decreasing_depth(list(range(1, 11)), length=5, max_depth=3) >>> assert data == [[[1, 2, 3, 4, 5], 6, 7, 8, 9, 10]] >>> data = create_data_decreasing_depth(list(range(1, 11)), length=2, max_depth=3) >>> assert data == [[[1, 2], 3, 4], 5, 6], [[7, 8,] 9, 10]]
create_data_increasing_depth
Implementaci贸n def create_data_increasing_depth( data: Union[List, Iterator], length: int, max_depth: int, _current_depth: int = None, _result: List = None ) -> List: """ creates data in depth to increase. Examples: >>> data = create_data_increasing_depth(list(range(1, 11)), length=5, max_depth=3) >>> assert data == [1, 2, 3, 4, 5, [6, 7, 8, 9, 10]] >>> data = create_data_increasing_depth(list(range(1, 11)), length=2, max_depth=3) >>> assert data == [1, 2, [3, 4, [5, 6]]], 7, 8, [9, 10]] """ _result = _result or [] _current_depth = _current_depth or max_depth data = iter(data) try: _current_length = length while _current_length: item = next(data) _result.append(item) _current_length -= 1 except StopIteration: return _result if _current_depth - 1: tmp_res = create_data_increasing_depth( data=data, length=length, max_depth=max_depth, _current_depth=_current_depth - 1) if tmp_res: _result.append(tmp_res) if max_depth == _current_depth: tmp_res = create_data_increasing_depth( data=data, length=length, max_depth=max_depth) if tmp_res: _result += tmp_res return _result
Esta funci贸n crea listas anidadas con anidamiento creciente.
>>> data = create_data_increasing_depth(list(range(1, 11)), length=5, max_depth=3) >>> assert data == [1, 2, 3, 4, 5, [6, 7, 8, 9, 10]] >>> data = create_data_increasing_depth(list(range(1, 11)), length=2, max_depth=3) >>> assert data == [1, 2, [3, 4, [5, 6]]], 7, 8, [9, 10]]
generar_datos
Implementaci贸n def generate_data() -> List[Tuple[str, Dict[str, Union[range, Num]]]]: """ Generated collections of Data by pattern {amount_item}_amount_{length}_length_{max_depth}_max_depth where: .. py:attribute:: amount_item: len of flatten elements .. py:attribute:: length: len of elements at the same level of nesting .. py:attribute:: max_depth: highest possible level of nesting """ data = [] amount_of_elements = [10 ** i for i in range(5)] data_template = '{amount_item}_amount_{length}_length_{max_depth}_max_depth'
Esta funci贸n crea directamente patrones para los datos de prueba. Para hacer esto, genera encabezados creados por la plantilla.
data_template = '{amount_item}_amount_{length}_length_{max_depth}_max_depth'
Donde:
- cantidad: el n煤mero total de elementos en la lista
- longitud: el n煤mero de elementos en un nivel de anidamiento
- max_depth: n煤mero m谩ximo de niveles de anidamiento
Los datos en s铆 se transfieren a las funciones anteriores para generar los datos necesarios. En consecuencia, la salida, como veremos m谩s adelante, tendremos los siguientes datos (encabezados):
10_amount_1_length_1_max_depth 100_amount_1_length_1_max_depth 100_amount_1_length_10_max_depth 100_amount_3_length_10_max_depth 100_amount_6_length_10_max_depth 100_amount_9_length_10_max_depth 1000_amount_1_length_1_max_depth 1000_amount_1_length_10_max_depth 1000_amount_3_length_10_max_depth 1000_amount_6_length_10_max_depth 1000_amount_9_length_10_max_depth 1000_amount_1_length_100_max_depth 1000_amount_25_length_100_max_depth 1000_amount_50_length_100_max_depth 1000_amount_75_length_100_max_depth 1000_amount_100_length_100_max_depth 10000_amount_1_length_1_max_depth 10000_amount_1_length_10_max_depth 10000_amount_3_length_10_max_depth 10000_amount_6_length_10_max_depth 10000_amount_9_length_10_max_depth 10000_amount_1_length_100_max_depth 10000_amount_25_length_100_max_depth 10000_amount_50_length_100_max_depth 10000_amount_75_length_100_max_depth 10000_amount_100_length_100_max_depth 10000_amount_1_length_1000_max_depth 10000_amount_250_length_1000_max_depth 10000_amount_500_length_1000_max_depth 10000_amount_750_length_1000_max_depth 10000_amount_1000_length_1000_max_depth
Parte 3. Resultados
Para perfilar la CPU, utilic茅 line_profiler
Para graficar - timeit + matplotlib
CPU Profiler
Conclusi贸n $ kernprof -l funcs.py $ python -m line_profiler funcs.py.lprof Timer unit: 1e-06 s Total time: 1.7e-05 s File: funcs.py Function: outer_flatten_1 at line 11 Line # Hits Time Per Hit % Time Line Contents ============================================================== 11 @profile 12 def outer_flatten_1(array: Iterable) -> List: 13 """ 14 Based on C realization of this solution 15 More on: 16 17 https://iteration-utilities.readthedocs.io/en/latest/generated/deepflatten.html 18 19 https://github.com/MSeifert04/iteration_utilities/blob/384948b4e82e41de47fa79fb73efc56c08549b01/src/deepflatten.c 20 """ 21 2 17.0 8.5 100.0 return deepflatten(array) Total time: 3.3e-05 s File: funcs.py Function: outer_flatten_2 at line 24 Line # Hits Time Per Hit % Time Line Contents ============================================================== 24 @profile 25 def outer_flatten_2(array: Iterable) -> List: 26 """ 27 recursive algorithm, vaguely reminiscent of recursion_flatten. Based on next pattern: 28 29 .. code:: python 30 31 try: 32 tree = iter(node) 33 except TypeError: 34 yield node 35 36 more on: 37 https://more-itertools.readthedocs.io/en/stable/api.html#more_itertools.collapse 38 """ 39 2 33.0 16.5 100.0 return collapse(array) Total time: 0.105099 s File: funcs.py Function: niccolum_flatten at line 42 Line # Hits Time Per Hit % Time Line Contents ============================================================== 42 @profile 43 def niccolum_flatten(array: Iterable) -> List: 44 """ 45 Non recursive algorithm 46 Based on pop/insert elements in current list 47 """ 48 49 2 39.0 19.5 0.0 new_array = array[:] 50 2 6.0 3.0 0.0 ind = 0 51 2 2.0 1.0 0.0 while True: 52 20002 7778.0 0.4 7.4 try: 53 21010 13528.0 0.6 12.9 while isinstance(new_array[ind], list): 54 1008 1520.0 1.5 1.4 item = new_array.pop(ind) 55 21014 13423.0 0.6 12.8 for inner_item in reversed(item): 56 20006 59375.0 3.0 56.5 new_array.insert(ind, inner_item) 57 20000 9423.0 0.5 9.0 ind += 1 58 2 2.0 1.0 0.0 except IndexError: 59 2 2.0 1.0 0.0 break 60 2 1.0 0.5 0.0 return new_array Total time: 0.137481 s File: funcs.py Function: tishka_flatten at line 63 Line # Hits Time Per Hit % Time Line Contents ============================================================== 63 @profile 64 def tishka_flatten(data: Iterable) -> List: 65 """ 66 Non recursive algorithm 67 Based on append/extend elements to new list 68 69 """ 70 2 17.0 8.5 0.0 nested = True 71 1012 1044.0 1.0 0.8 while nested: 72 1010 1063.0 1.1 0.8 new = [] 73 1010 992.0 1.0 0.7 nested = False 74 112018 38090.0 0.3 27.7 for i in data: 75 111008 50247.0 0.5 36.5 if isinstance(i, list): 76 1008 1431.0 1.4 1.0 new.extend(i) 77 1008 1138.0 1.1 0.8 nested = True 78 else: 79 110000 42052.0 0.4 30.6 new.append(i) 80 1010 1406.0 1.4 1.0 data = new 81 2 1.0 0.5 0.0 return data Total time: 0.062931 s File: funcs.py Function: zart_flatten at line 84 Line # Hits Time Per Hit % Time Line Contents ============================================================== 84 @profile 85 def zart_flatten(a: Iterable) -> List: 86 """ 87 Non recursive algorithm 88 Based on pop from old and append elements to new list 89 """ 90 2 20.0 10.0 0.0 queue, out = [a], [] 91 21012 12866.0 0.6 20.4 while queue: 92 21010 16849.0 0.8 26.8 elem = queue.pop(-1) 93 21010 17768.0 0.8 28.2 if isinstance(elem, list): 94 1010 1562.0 1.5 2.5 queue.extend(elem) 95 else: 96 20000 13813.0 0.7 21.9 out.append(elem) 97 2 53.0 26.5 0.1 return out[::-1] Total time: 0.052754 s File: funcs.py Function: recursive_flatten_generator at line 100 Line # Hits Time Per Hit % Time Line Contents ============================================================== 100 @profile 101 def recursive_flatten_generator(array: Iterable) -> List: 102 """ 103 Recursive algorithm 104 Looks like recursive_flatten_iterator, but with extend/append 105 106 """ 107 1010 1569.0 1.6 3.0 lst = [] 108 22018 13565.0 0.6 25.7 for i in array: 109 21008 17060.0 0.8 32.3 if isinstance(i, list): 110 1008 6624.0 6.6 12.6 lst.extend(recursive_flatten_generator(i)) 111 else: 112 20000 13622.0 0.7 25.8 lst.append(i) 113 1010 314.0 0.3 0.6 return lst Total time: 0.054103 s File: funcs.py Function: recursive_flatten_iterator at line 116 Line # Hits Time Per Hit % Time Line Contents ============================================================== 116 @profile 117 def recursive_flatten_iterator(arr: Iterable) -> Iterator: 118 """ 119 Recursive algorithm based on iterator 120 Usual solution to this problem 121 122 """ 123 124 22018 20200.0 0.9 37.3 for i in arr: 125 21008 19363.0 0.9 35.8 if isinstance(i, list): 126 1008 6856.0 6.8 12.7 yield from recursive_flatten_iterator(i) 127 else: 128 20000 7684.0 0.4 14.2 yield i Total time: 0.056111 s File: funcs.py Function: tishka_flatten_with_stack at line 131 Line # Hits Time Per Hit % Time Line Contents ============================================================== 131 @profile 132 def tishka_flatten_with_stack(seq: Iterable) -> List: 133 """ 134 Non recursive algorithm 135 Based on zart_flatten, but build on try/except pattern 136 """ 137 2 24.0 12.0 0.0 stack = [iter(seq)] 138 2 5.0 2.5 0.0 new = [] 139 1012 357.0 0.4 0.6 while stack: 140 1010 435.0 0.4 0.8 i = stack.pop() 141 1010 328.0 0.3 0.6 try: 142 1010 330.0 0.3 0.6 while True: 143 22018 17272.0 0.8 30.8 data = next(i) 144 21008 18951.0 0.9 33.8 if isinstance(data, list): 145 1008 997.0 1.0 1.8 stack.append(i) 146 1008 1205.0 1.2 2.1 i = iter(data) 147 else: 148 20000 15413.0 0.8 27.5 new.append(data) 149 1010 425.0 0.4 0.8 except StopIteration: 150 1010 368.0 0.4 0.7 pass 151 2 1.0 0.5 0.0 return new
Gr谩ficos
Resultado general:
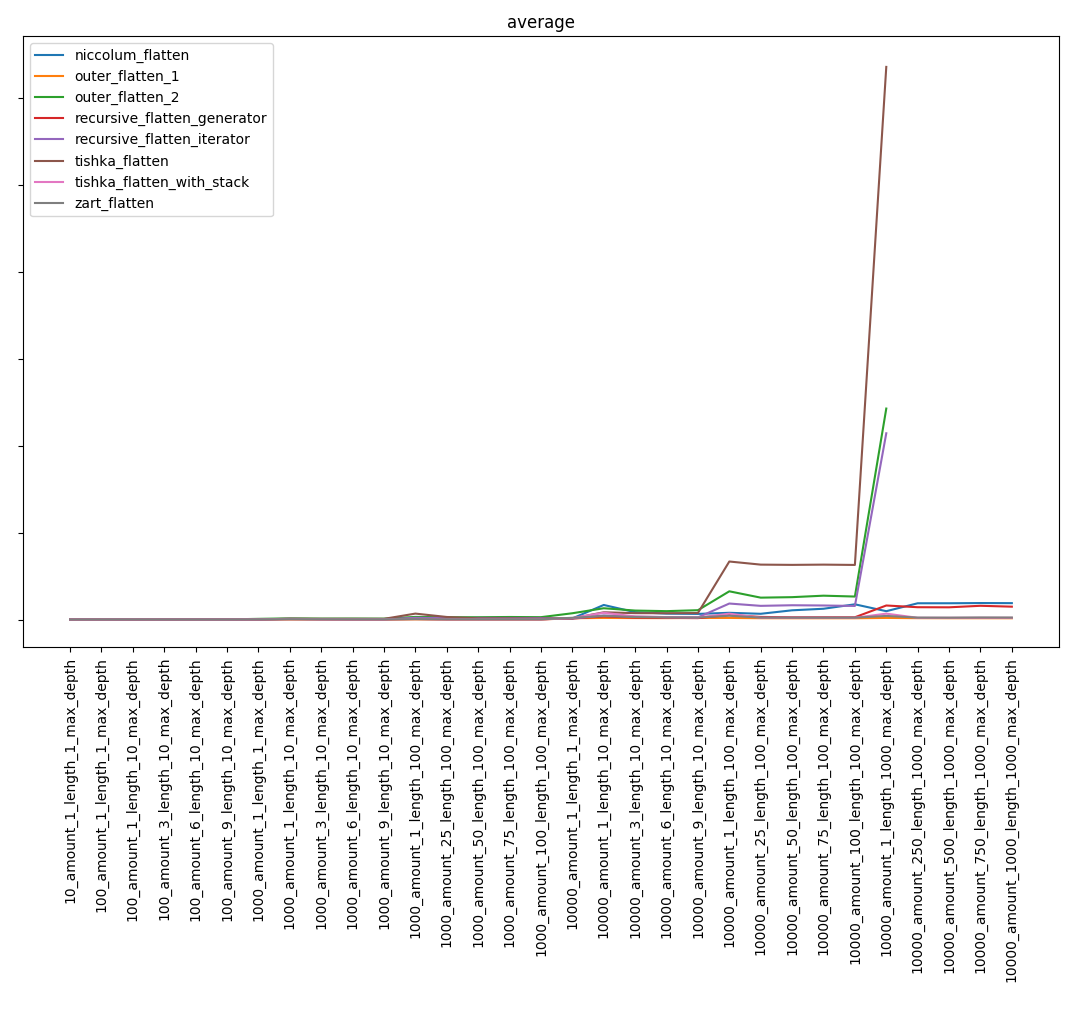
Excluyendo las funciones m谩s lentas, obtenemos:
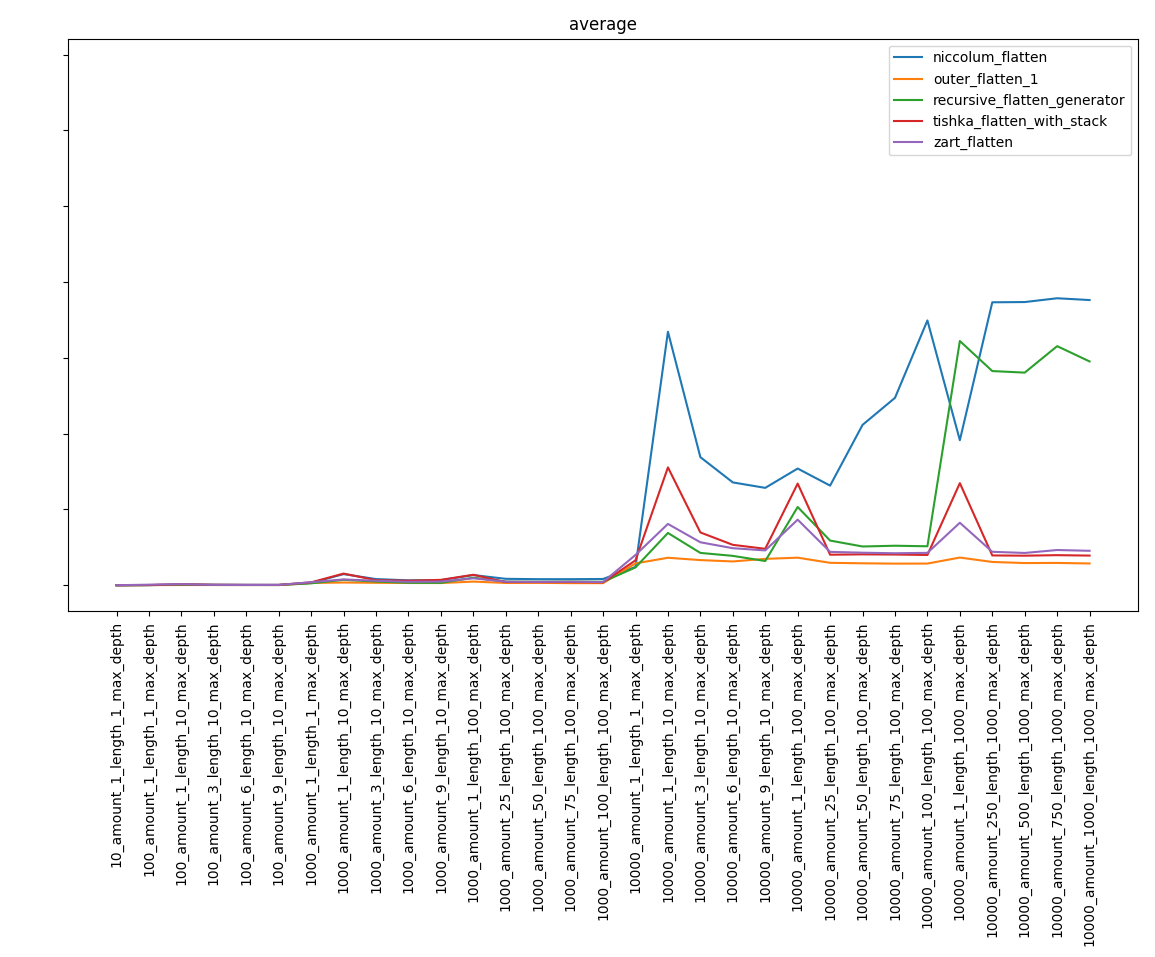
Parte 4. Conclusiones
Quiz谩s dir茅 lo obvio, pero, a pesar de la complejidad conocida de los algoritmos, el resultado puede no ser obvio. Entonces, la funci贸n niccolum_flatten, cuya complejidad era la m谩s alta, entr贸 en la etapa final y se alej贸 del 煤ltimo lugar. Y recursive_flatten_generator result贸 ser mucho m谩s r谩pido que recursive_flatten_iterator.
En resumen, quiero decir en primer lugar que esto fue m谩s un estudio que una gu铆a para escribir algoritmos efectivos de desempaquetado de listas. Por lo general, puede escribir la implementaci贸n m谩s simple sin pensar si es la m谩s r谩pida, porque Peque帽os ahorros.
Enlaces utiles
Se pueden encontrar resultados m谩s completos aqu铆.
Repositorio con c贸digo aqu铆
Documentaci贸n v铆a esfinge aqu铆
Si encuentra alg煤n error, escriba al telegrama de Niccolum o a lastsal@mail.ru.
Estar茅 contento con la cr铆tica constructiva.