Hoje eu gostaria de falar sobre como descompactar listas aninhadas de profundidade indefinida. Essa é uma tarefa bastante trivial, então eu gostaria de dizer aqui sobre o que são implementações, seus prós e contras e uma comparação de seu desempenho.
O artigo consistirá em várias seções abaixo:
- Funções
- Dados
- Resultados
- Conclusões
Parte 1. Funções
Implementações emprestadas
outer_flatten_1
Implementaçãodef outer_flatten_1(array: Iterable) -> List: """ Based on C realization of this solution More on: https://iteration-utilities.readthedocs.io/en/latest/generated/deepflatten.html https://github.com/MSeifert04/iteration_utilities/blob/384948b4e82e41de47fa79fb73efc56c08549b01/src/deepflatten.c """ return deepflatten(array)
Eu peguei essa função para analisar de um pacote externo, iteration_utilities.
A implementação foi feita em C, deixando para o python uma interface de chamada de função de alto nível.
A implementação da função em C é bastante complicada, você pode vê-la clicando no link no spoiler. A função é um iterador.
>>> from typing import Iterator, Generator >>> from iteration_utilities import deepflatten >>> isinstance(deepflatten(a), Iterator) True >>> isinstance(deepflatten(a), Generator) False
É difícil dizer sobre a complexidade do algoritmo implementado aqui, por isso deixo esse interesse para os usuários do Habr.
Em geral, eu também gostaria de observar que todas as outras funções desta biblioteca são bastante rápidas e também são implementadas em C.
outer_flatten_2
Implementação def outer_flatten_2(array: Iterable) -> List: """ recursive algorithm, vaguely reminiscent of recursion_flatten. Based on next pattern: .. code:: python try: tree = iter(node) except TypeError: yield node more on: https://more-itertools.readthedocs.io/en/stable/api.html#more_itertools.collapse """ return collapse(array)
A implementação desse método de descompactar listas aninhadas também está no pacote externo, ou seja, more_itertools.
A função é executada em python puro, mas não ideal, na minha opinião. Uma implementação detalhada pode ser vista no link da documentação.
A complexidade desse algoritmo é O (n * m).
Implementações próprias
niccolum_flatten
Implementação def niccolum_flatten(array: Iterable) -> List: """ Non recursive algorithm Based on pop/insert elements in current list """ new_array = array[:] ind = 0 while True: try: while isinstance(new_array[ind], list): item = new_array.pop(ind) for inner_item in reversed(item): new_array.insert(ind, inner_item) ind += 1 except IndexError: break return new_array
Quando, de alguma maneira, falei sobre telegramas ao público @ru_python_beginners sobre a implementação de descompactar listas aninhadas de aninhamento indefinido, propus minha própria versão.
Consiste no fato de copiarmos a lista original (para não alterá-la) e, no loop while True, verificamos enquanto o item é uma lista - passamos por ela e inserimos o resultado no início.
Sim, agora entendo que não parece ótimo e difícil, porque a reindexação ocorre a cada vez (desde que adicionamos e removemos do topo da lista), no entanto, essa opção também tem direito à vida e verificaremos sua implementação junto com o restante.
A complexidade desse algoritmo é O (n ^ 3 * m) devido à reconstrução da lista duas vezes para cada iteração passada
tishka_flatten
Implementação def tishka_flatten(data: Iterable) -> List: """ Non recursive algorithm Based on append/extend elements to new list """ nested = True while nested: new = [] nested = False for i in data: if isinstance(i, list): new.extend(i) nested = True else: new.append(i) data = new return data
Um dos primeiros também mostrou a implementação do Tishka17 . Consiste no fato de que, dentro do loop aninhado while, itera sobre a lista existente com a chave aninhada = False, e se pelo menos uma vez obteve uma planilha, ela deixa o sinalizador aninhado = True e a estende à planilha. Consequentemente, verifica-se que, para cada execução, estabelece um nível de aninhamento, quantos níveis de aninhamento haverá - haverá tantas passagens pelo ciclo. Como pode ser visto na descrição - não é o algoritmo mais ideal, mas ainda assim, é diferente do resto.
A complexidade desse algoritmo é O (n * m).
zart_flatten
Implementação def zart_flatten(a: Iterable) -> List: """ Non recursive algorithm Based on pop from old and append elements to new list """ queue, out = [a], [] while queue: elem = queue.pop(-1) if isinstance(elem, list): queue.extend(elem) else: out.append(elem) return out[::-1]
Um algoritmo também sugerido por um dos participantes experientes do chat. Na minha opinião, é bastante simples e limpo. Seu significado é que, se o elemento for uma lista, adicionamos o resultado ao final da lista original e, se não, o adicionamos à saída. Vou chamá-lo abaixo do mecanismo de extensão / adição. Como resultado, exibimos a lista plana resultante resultante invertida para preservar a ordem original dos elementos.
A complexidade desse algoritmo é O (n * m).
recursive_flatten_iterator
Implementação def recursive_flatten_iterator(arr: Iterable) -> Iterator: """ Recursive algorithm based on iterator Usual solution to this problem """ for i in arr: if isinstance(i, list): yield from recursion_flatten(i) else: yield i
Provavelmente, a solução mais comum para esse problema é através da recursão e rendimento de. Pouco se pode dizer - o algoritmo parece bastante simples e eficiente, além do fato de ser feito por recursão e, com aninhamento grande, pode haver uma pilha bastante grande de chamadas.
A complexidade desse algoritmo é O (n * m).
recursive_flatten_generator
Implementação def recursive_flatten_generator(array: Iterable) -> List: """ Recursive algorithm Looks like recursive_flatten_iterator, but with extend/append """ lst = [] for i in array: if isinstance(i, list): lst.extend(recursive_flatten_list(i)) else: lst.append(i) return lst
Esta função é bastante semelhante à anterior, sendo executada apenas não através de um iterador, mas através de um mecanismo recursivo de extensão / adição.
A complexidade desse algoritmo é O (n * m).
tishka_flatten_with_stack
Implementação def tishka_flatten_with_stack(seq: Iterable) -> List: """ Non recursive algorithm Based on zart_flatten, but build on try/except pattern """ stack = [iter(seq)] new = [] while stack: i = stack.pop() try: while True: data = next(i) if isinstance(data, list): stack.append(i) i = iter(data) else: new.append(data) except StopIteration: pass return new
Mais um algoritmo que o Tishka forneceu, o qual, de fato, é muito semelhante ao zart_flatten, no entanto, foi implementado por meio de um mecanismo simples de extensão / adição, depois pela iteração em um loop infinito no qual esse mecanismo é usado. Assim, resultou algo semelhante ao zart_flatten, mas através da iteração e enquanto True.
A complexidade desse algoritmo é O (n * m).
Parte 2. Dados
Para testar a eficácia desses algoritmos, precisamos criar funções para a geração automática de listas de um determinado aninhamento, com as quais eu lidei com sucesso e mostrarei o resultado abaixo:
create_data_decreasing_depth
Implementação def create_data_decreasing_depth( data: Union[List, Iterator], length: int, max_depth: int, _current_depth: int = None, _result: List = None ) -> List: """ creates data in depth on decreasing. Examples: >>> data = create_data_decreasing_depth(list(range(1, 11)), length=5, max_depth=3) >>> assert data == [[[1, 2, 3, 4, 5], 6, 7, 8, 9, 10]] >>> data = create_data_decreasing_depth(list(range(1, 11)), length=2, max_depth=3) >>> assert data == [[[1, 2], 3, 4], 5, 6], [[7, 8,] 9, 10]] """ _result = _result or [] _current_depth = _current_depth or max_depth data = iter(data) if _current_depth - 1: _result.append(create_data_decreasing_depth( data=data, length=length, max_depth=max_depth, _current_depth=_current_depth - 1, _result=_result)) try: _current_length = length while _current_length: item = next(data) _result.append(item) _current_length -= 1 if max_depth == _current_depth: _result += create_data_decreasing_depth( data=data, length=length, max_depth=max_depth) return _result except StopIteration: return _result
Esta função cria listas aninhadas com aninhamento decrescente.
>>> data = create_data_decreasing_depth(list(range(1, 11)), length=5, max_depth=3) >>> assert data == [[[1, 2, 3, 4, 5], 6, 7, 8, 9, 10]] >>> data = create_data_decreasing_depth(list(range(1, 11)), length=2, max_depth=3) >>> assert data == [[[1, 2], 3, 4], 5, 6], [[7, 8,] 9, 10]]
create_data_increasing_depth
Implementação def create_data_increasing_depth( data: Union[List, Iterator], length: int, max_depth: int, _current_depth: int = None, _result: List = None ) -> List: """ creates data in depth to increase. Examples: >>> data = create_data_increasing_depth(list(range(1, 11)), length=5, max_depth=3) >>> assert data == [1, 2, 3, 4, 5, [6, 7, 8, 9, 10]] >>> data = create_data_increasing_depth(list(range(1, 11)), length=2, max_depth=3) >>> assert data == [1, 2, [3, 4, [5, 6]]], 7, 8, [9, 10]] """ _result = _result or [] _current_depth = _current_depth or max_depth data = iter(data) try: _current_length = length while _current_length: item = next(data) _result.append(item) _current_length -= 1 except StopIteration: return _result if _current_depth - 1: tmp_res = create_data_increasing_depth( data=data, length=length, max_depth=max_depth, _current_depth=_current_depth - 1) if tmp_res: _result.append(tmp_res) if max_depth == _current_depth: tmp_res = create_data_increasing_depth( data=data, length=length, max_depth=max_depth) if tmp_res: _result += tmp_res return _result
Essa função cria listas aninhadas com aumento do aninhamento.
>>> data = create_data_increasing_depth(list(range(1, 11)), length=5, max_depth=3) >>> assert data == [1, 2, 3, 4, 5, [6, 7, 8, 9, 10]] >>> data = create_data_increasing_depth(list(range(1, 11)), length=2, max_depth=3) >>> assert data == [1, 2, [3, 4, [5, 6]]], 7, 8, [9, 10]]
generate_data
Implementação def generate_data() -> List[Tuple[str, Dict[str, Union[range, Num]]]]: """ Generated collections of Data by pattern {amount_item}_amount_{length}_length_{max_depth}_max_depth where: .. py:attribute:: amount_item: len of flatten elements .. py:attribute:: length: len of elements at the same level of nesting .. py:attribute:: max_depth: highest possible level of nesting """ data = [] amount_of_elements = [10 ** i for i in range(5)] data_template = '{amount_item}_amount_{length}_length_{max_depth}_max_depth'
Esta função cria diretamente padrões para dados de teste. Para fazer isso, ele gera cabeçalhos criados pelo modelo.
data_template = '{amount_item}_amount_{length}_length_{max_depth}_max_depth'
Onde:
- quantidade - o número total de itens na lista
- length - o número de elementos em um nível de aninhamento
- max_depth - número máximo de níveis de aninhamento
Os dados em si são transferidos para as funções acima para gerar os dados necessários. Assim, a saída, como veremos mais adiante, teremos os seguintes dados (cabeçalhos):
10_amount_1_length_1_max_depth 100_amount_1_length_1_max_depth 100_amount_1_length_10_max_depth 100_amount_3_length_10_max_depth 100_amount_6_length_10_max_depth 100_amount_9_length_10_max_depth 1000_amount_1_length_1_max_depth 1000_amount_1_length_10_max_depth 1000_amount_3_length_10_max_depth 1000_amount_6_length_10_max_depth 1000_amount_9_length_10_max_depth 1000_amount_1_length_100_max_depth 1000_amount_25_length_100_max_depth 1000_amount_50_length_100_max_depth 1000_amount_75_length_100_max_depth 1000_amount_100_length_100_max_depth 10000_amount_1_length_1_max_depth 10000_amount_1_length_10_max_depth 10000_amount_3_length_10_max_depth 10000_amount_6_length_10_max_depth 10000_amount_9_length_10_max_depth 10000_amount_1_length_100_max_depth 10000_amount_25_length_100_max_depth 10000_amount_50_length_100_max_depth 10000_amount_75_length_100_max_depth 10000_amount_100_length_100_max_depth 10000_amount_1_length_1000_max_depth 10000_amount_250_length_1000_max_depth 10000_amount_500_length_1000_max_depth 10000_amount_750_length_1000_max_depth 10000_amount_1000_length_1000_max_depth
Parte 3. Resultados
Para criar o perfil da CPU - usei line_profiler
Para gráficos - timeit + matplotlib
CPU Profiler
Conclusão $ kernprof -l funcs.py $ python -m line_profiler funcs.py.lprof Timer unit: 1e-06 s Total time: 1.7e-05 s File: funcs.py Function: outer_flatten_1 at line 11 Line # Hits Time Per Hit % Time Line Contents ============================================================== 11 @profile 12 def outer_flatten_1(array: Iterable) -> List: 13 """ 14 Based on C realization of this solution 15 More on: 16 17 https://iteration-utilities.readthedocs.io/en/latest/generated/deepflatten.html 18 19 https://github.com/MSeifert04/iteration_utilities/blob/384948b4e82e41de47fa79fb73efc56c08549b01/src/deepflatten.c 20 """ 21 2 17.0 8.5 100.0 return deepflatten(array) Total time: 3.3e-05 s File: funcs.py Function: outer_flatten_2 at line 24 Line # Hits Time Per Hit % Time Line Contents ============================================================== 24 @profile 25 def outer_flatten_2(array: Iterable) -> List: 26 """ 27 recursive algorithm, vaguely reminiscent of recursion_flatten. Based on next pattern: 28 29 .. code:: python 30 31 try: 32 tree = iter(node) 33 except TypeError: 34 yield node 35 36 more on: 37 https://more-itertools.readthedocs.io/en/stable/api.html#more_itertools.collapse 38 """ 39 2 33.0 16.5 100.0 return collapse(array) Total time: 0.105099 s File: funcs.py Function: niccolum_flatten at line 42 Line # Hits Time Per Hit % Time Line Contents ============================================================== 42 @profile 43 def niccolum_flatten(array: Iterable) -> List: 44 """ 45 Non recursive algorithm 46 Based on pop/insert elements in current list 47 """ 48 49 2 39.0 19.5 0.0 new_array = array[:] 50 2 6.0 3.0 0.0 ind = 0 51 2 2.0 1.0 0.0 while True: 52 20002 7778.0 0.4 7.4 try: 53 21010 13528.0 0.6 12.9 while isinstance(new_array[ind], list): 54 1008 1520.0 1.5 1.4 item = new_array.pop(ind) 55 21014 13423.0 0.6 12.8 for inner_item in reversed(item): 56 20006 59375.0 3.0 56.5 new_array.insert(ind, inner_item) 57 20000 9423.0 0.5 9.0 ind += 1 58 2 2.0 1.0 0.0 except IndexError: 59 2 2.0 1.0 0.0 break 60 2 1.0 0.5 0.0 return new_array Total time: 0.137481 s File: funcs.py Function: tishka_flatten at line 63 Line # Hits Time Per Hit % Time Line Contents ============================================================== 63 @profile 64 def tishka_flatten(data: Iterable) -> List: 65 """ 66 Non recursive algorithm 67 Based on append/extend elements to new list 68 69 """ 70 2 17.0 8.5 0.0 nested = True 71 1012 1044.0 1.0 0.8 while nested: 72 1010 1063.0 1.1 0.8 new = [] 73 1010 992.0 1.0 0.7 nested = False 74 112018 38090.0 0.3 27.7 for i in data: 75 111008 50247.0 0.5 36.5 if isinstance(i, list): 76 1008 1431.0 1.4 1.0 new.extend(i) 77 1008 1138.0 1.1 0.8 nested = True 78 else: 79 110000 42052.0 0.4 30.6 new.append(i) 80 1010 1406.0 1.4 1.0 data = new 81 2 1.0 0.5 0.0 return data Total time: 0.062931 s File: funcs.py Function: zart_flatten at line 84 Line # Hits Time Per Hit % Time Line Contents ============================================================== 84 @profile 85 def zart_flatten(a: Iterable) -> List: 86 """ 87 Non recursive algorithm 88 Based on pop from old and append elements to new list 89 """ 90 2 20.0 10.0 0.0 queue, out = [a], [] 91 21012 12866.0 0.6 20.4 while queue: 92 21010 16849.0 0.8 26.8 elem = queue.pop(-1) 93 21010 17768.0 0.8 28.2 if isinstance(elem, list): 94 1010 1562.0 1.5 2.5 queue.extend(elem) 95 else: 96 20000 13813.0 0.7 21.9 out.append(elem) 97 2 53.0 26.5 0.1 return out[::-1] Total time: 0.052754 s File: funcs.py Function: recursive_flatten_generator at line 100 Line # Hits Time Per Hit % Time Line Contents ============================================================== 100 @profile 101 def recursive_flatten_generator(array: Iterable) -> List: 102 """ 103 Recursive algorithm 104 Looks like recursive_flatten_iterator, but with extend/append 105 106 """ 107 1010 1569.0 1.6 3.0 lst = [] 108 22018 13565.0 0.6 25.7 for i in array: 109 21008 17060.0 0.8 32.3 if isinstance(i, list): 110 1008 6624.0 6.6 12.6 lst.extend(recursive_flatten_generator(i)) 111 else: 112 20000 13622.0 0.7 25.8 lst.append(i) 113 1010 314.0 0.3 0.6 return lst Total time: 0.054103 s File: funcs.py Function: recursive_flatten_iterator at line 116 Line # Hits Time Per Hit % Time Line Contents ============================================================== 116 @profile 117 def recursive_flatten_iterator(arr: Iterable) -> Iterator: 118 """ 119 Recursive algorithm based on iterator 120 Usual solution to this problem 121 122 """ 123 124 22018 20200.0 0.9 37.3 for i in arr: 125 21008 19363.0 0.9 35.8 if isinstance(i, list): 126 1008 6856.0 6.8 12.7 yield from recursive_flatten_iterator(i) 127 else: 128 20000 7684.0 0.4 14.2 yield i Total time: 0.056111 s File: funcs.py Function: tishka_flatten_with_stack at line 131 Line # Hits Time Per Hit % Time Line Contents ============================================================== 131 @profile 132 def tishka_flatten_with_stack(seq: Iterable) -> List: 133 """ 134 Non recursive algorithm 135 Based on zart_flatten, but build on try/except pattern 136 """ 137 2 24.0 12.0 0.0 stack = [iter(seq)] 138 2 5.0 2.5 0.0 new = [] 139 1012 357.0 0.4 0.6 while stack: 140 1010 435.0 0.4 0.8 i = stack.pop() 141 1010 328.0 0.3 0.6 try: 142 1010 330.0 0.3 0.6 while True: 143 22018 17272.0 0.8 30.8 data = next(i) 144 21008 18951.0 0.9 33.8 if isinstance(data, list): 145 1008 997.0 1.0 1.8 stack.append(i) 146 1008 1205.0 1.2 2.1 i = iter(data) 147 else: 148 20000 15413.0 0.8 27.5 new.append(data) 149 1010 425.0 0.4 0.8 except StopIteration: 150 1010 368.0 0.4 0.7 pass 151 2 1.0 0.5 0.0 return new
Gráficos
Resultado geral:
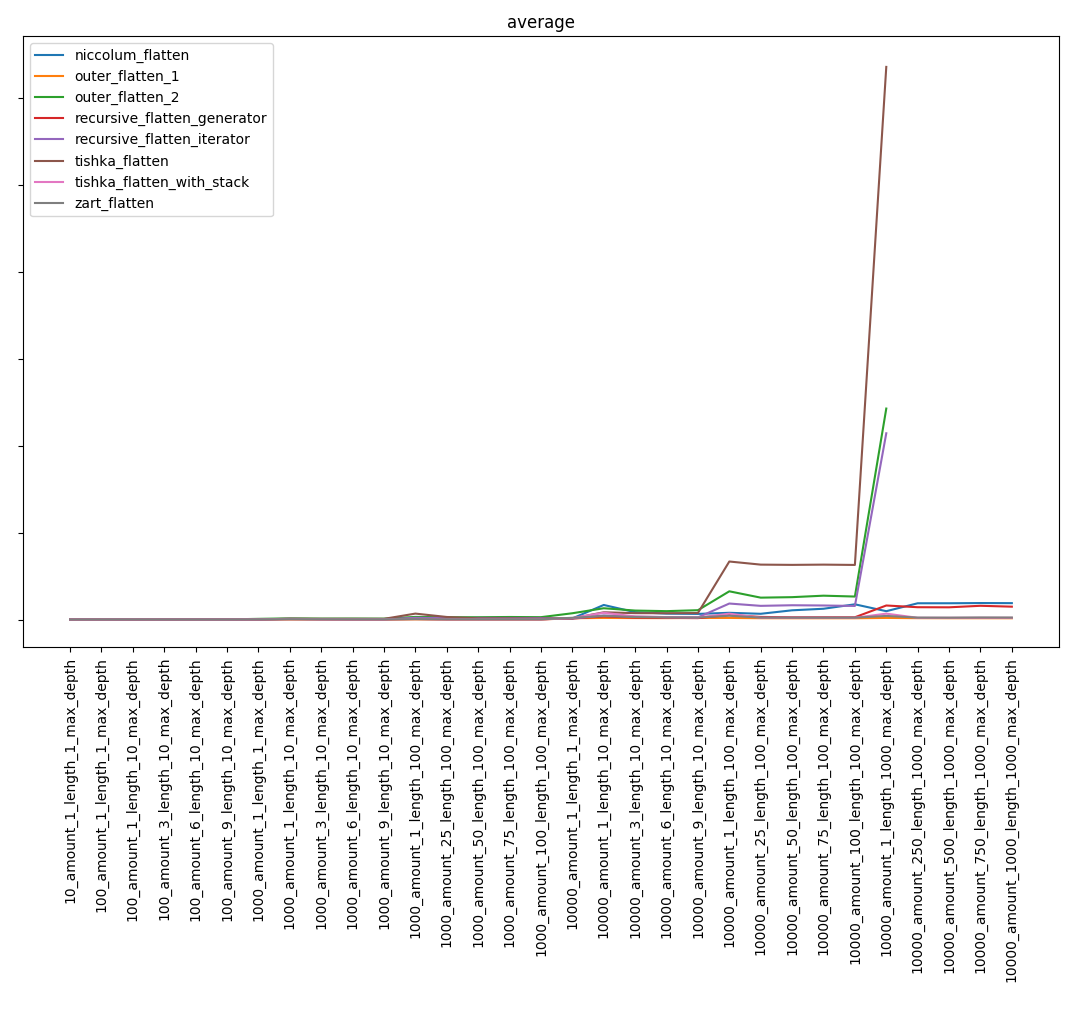
Excluindo as funções mais lentas, obtemos:
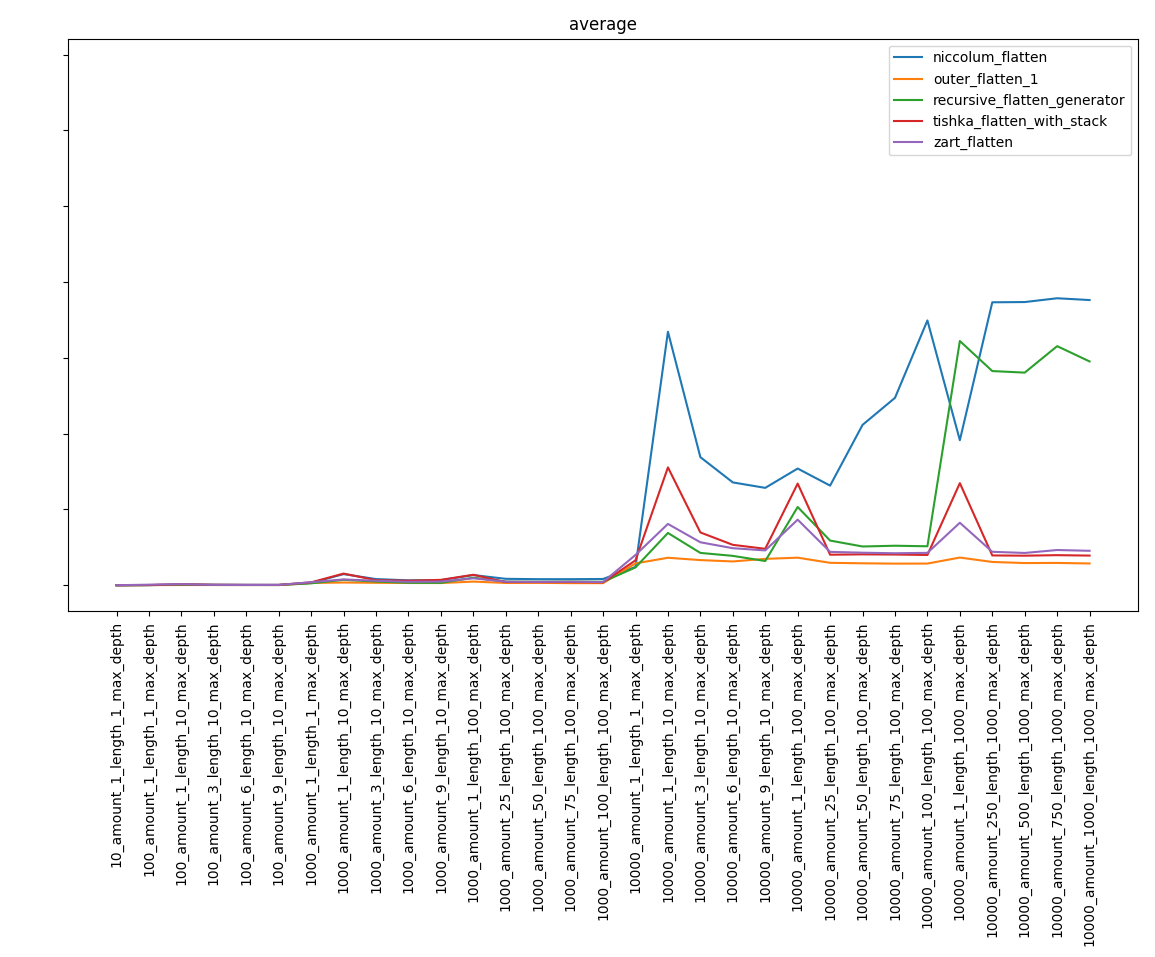
Parte 4. Conclusões
Talvez eu diga o óbvio, mas, apesar da complexidade conhecida dos algoritmos, o resultado pode não ser óbvio. Portanto, a função niccolum_flatten, cuja complexidade era mais alta, entrou na fase final e ficou longe do último lugar. E recursive_flatten_generator acabou sendo muito mais rápido que recursive_flatten_iterator.
Resumindo, quero dizer antes de tudo que isso foi mais um estudo do que um guia para escrever algoritmos eficazes de descompactação de lista. Geralmente, você pode escrever a implementação mais simples sem pensar se é a mais rápida, porque poucas economias.
Links úteis
Resultados mais completos podem ser encontrados aqui.
Repositório com código aqui
Documentação via sphinx aqui
Se encontrar algum erro, escreva para o telegrama Niccolum ou para lastsal@mail.ru.
Ficarei feliz em receber críticas construtivas.