今天,我想谈谈解开不确定深度的嵌套列表。 这是一项不平凡的任务,所以我想在这里谈谈什么是实现,它们的优缺点以及它们的性能比较。
本文将由以下几部分组成:
第1部分。功能
借用的实现
outside_flatten_1
实作def outer_flatten_1(array: Iterable) -> List: """ Based on C realization of this solution More on: https://iteration-utilities.readthedocs.io/en/latest/generated/deepflatten.html https://github.com/MSeifert04/iteration_utilities/blob/384948b4e82e41de47fa79fb73efc56c08549b01/src/deepflatten.c """ return deepflatten(array)
我使用此函数从外部程序包eration_utilities进行解析。
该实现是用C语言完成的,为python留下了一个高级函数调用接口。
在C中该功能的实现相当麻烦,您可以通过单击扰流板中的链接来查看它。 该函数是一个迭代器。
>>> from typing import Iterator, Generator >>> from iteration_utilities import deepflatten >>> isinstance(deepflatten(a), Iterator) True >>> isinstance(deepflatten(a), Generator) False
关于此处实现的算法的复杂性很难说,因此我将这种兴趣留给了Habr用户。
总的来说,我还要指出,该库中的所有其他函数都非常快,并且也用C实现。
outside_flatten_2
实作 def outer_flatten_2(array: Iterable) -> List: """ recursive algorithm, vaguely reminiscent of recursion_flatten. Based on next pattern: .. code:: python try: tree = iter(node) except TypeError: yield node more on: https://more-itertools.readthedocs.io/en/stable/api.html#more_itertools.collapse """ return collapse(array)
这种解压缩嵌套列表的方法的实现也在外部包中,即more_itertools。
我认为该功能是在纯python上执行的,但不是最佳的。 详细的实现方式可以在文档链接中找到 。
该算法的复杂度为O(n * m)。
自己的实现
niccolum_flatten
实作 def niccolum_flatten(array: Iterable) -> List: """ Non recursive algorithm Based on pop/insert elements in current list """ new_array = array[:] ind = 0 while True: try: while isinstance(new_array[ind], list): item = new_array.pop(ind) for inner_item in reversed(item): new_array.insert(ind, inner_item) ind += 1 except IndexError: break return new_array
当以某种方式向公众@ru_python_beginners发出电报时,涉及到解包不确定嵌套的嵌套列表的实现时,我提出了自己的选择。
它包含以下事实:我们复制原始列表(以免更改它),然后在while True循环中检查项目是列表时-我们遍历并将结果插入到最开始。
是的,现在我知道它看起来并不理想且困难,因为 每次都会进行重新索引编制(因为我们从列表的顶部进行添加和删除),但是此选项也具有生命权,我们将与其他选项一起检查其实现。
该算法的复杂度为O(n ^ 3 * m),这是因为每次传递的迭代都两次重建了列表
tishka_flatten
实作 def tishka_flatten(data: Iterable) -> List: """ Non recursive algorithm Based on append/extend elements to new list """ nested = True while nested: new = [] nested = False for i in data: if isinstance(i, list): new.extend(i) nested = True else: new.append(i) data = new return data
第一个也显示了Tishka17的实现。 它包含以下事实:while嵌套循环内部使用键nested = False遍历现有列表,如果至少有一次获取工作表,它将保留nested = True标志并将此工作表扩展到列表。 相应地,事实证明,对于每个运行,它都会安排一个嵌套级别,即将嵌套多少级别-整个循环中会有很多通过。 从描述中可以看出-不是最佳算法,但是与其他算法有所不同。
该算法的复杂度为O(n * m)。
zart_flatten
实作 def zart_flatten(a: Iterable) -> List: """ Non recursive algorithm Based on pop from old and append elements to new list """ queue, out = [a], [] while queue: elem = queue.pop(-1) if isinstance(elem, list): queue.extend(elem) else: out.append(elem) return out[::-1]
经验丰富的聊天参与者之一也提出了一种算法。 我认为它非常简单和干净。 它的含义是,如果元素是列表,则将结果添加到原始列表的末尾,如果不是,则将其添加到输出。 我将在扩展/追加机制下称呼它。 结果,我们显示反转的结果平面图,以保留元素的原始顺序。
该算法的复杂度为O(n * m)。
recursive_flatten_iterator
实作 def recursive_flatten_iterator(arr: Iterable) -> Iterator: """ Recursive algorithm based on iterator Usual solution to this problem """ for i in arr: if isinstance(i, list): yield from recursion_flatten(i) else: yield i
解决此问题的最常见方法可能是通过递归和收益。 几乎没有什么可以说的-该算法看起来非常简单和高效,除了它是通过递归完成的,而且对于大型机箱而言,可能会有相当多的调用堆栈。
该算法的复杂度为O(n * m)。
recursive_flatten_generator
实作 def recursive_flatten_generator(array: Iterable) -> List: """ Recursive algorithm Looks like recursive_flatten_iterator, but with extend/append """ lst = [] for i in array: if isinstance(i, list): lst.extend(recursive_flatten_list(i)) else: lst.append(i) return lst
此功能与上一个功能非常相似,它不是通过迭代器执行的,而是通过递归扩展/追加机制执行的。
该算法的复杂度为O(n * m)。
tishka_flatten_with_stack
实作 def tishka_flatten_with_stack(seq: Iterable) -> List: """ Non recursive algorithm Based on zart_flatten, but build on try/except pattern """ stack = [iter(seq)] new = [] while stack: i = stack.pop() try: while True: data = next(i) if isinstance(data, list): stack.append(i) i = iter(data) else: new.append(data) except StopIteration: pass return new
Tishka提供的另一种算法,实际上与zart_flatten非常相似,但是,它是通过简单的扩展/附加机制在此处实现的,然后通过使用该机制的无限循环中的迭代来实现的。 因此,结果类似于zart_flatten,但经过迭代和True。
该算法的复杂度为O(n * m)。
第2部分。数据
为了测试这些算法的有效性,我们需要创建某些嵌套列表的自动生成功能,这些功能我已经成功进行了管理,并将在下面显示结果:
create_data_decreasing_depth
实作 def create_data_decreasing_depth( data: Union[List, Iterator], length: int, max_depth: int, _current_depth: int = None, _result: List = None ) -> List: """ creates data in depth on decreasing. Examples: >>> data = create_data_decreasing_depth(list(range(1, 11)), length=5, max_depth=3) >>> assert data == [[[1, 2, 3, 4, 5], 6, 7, 8, 9, 10]] >>> data = create_data_decreasing_depth(list(range(1, 11)), length=2, max_depth=3) >>> assert data == [[[1, 2], 3, 4], 5, 6], [[7, 8,] 9, 10]] """ _result = _result or [] _current_depth = _current_depth or max_depth data = iter(data) if _current_depth - 1: _result.append(create_data_decreasing_depth( data=data, length=length, max_depth=max_depth, _current_depth=_current_depth - 1, _result=_result)) try: _current_length = length while _current_length: item = next(data) _result.append(item) _current_length -= 1 if max_depth == _current_depth: _result += create_data_decreasing_depth( data=data, length=length, max_depth=max_depth) return _result except StopIteration: return _result
此函数使用减少的嵌套创建嵌套列表。
>>> data = create_data_decreasing_depth(list(range(1, 11)), length=5, max_depth=3) >>> assert data == [[[1, 2, 3, 4, 5], 6, 7, 8, 9, 10]] >>> data = create_data_decreasing_depth(list(range(1, 11)), length=2, max_depth=3) >>> assert data == [[[1, 2], 3, 4], 5, 6], [[7, 8,] 9, 10]]
create_data_increasing_depth
实作 def create_data_increasing_depth( data: Union[List, Iterator], length: int, max_depth: int, _current_depth: int = None, _result: List = None ) -> List: """ creates data in depth to increase. Examples: >>> data = create_data_increasing_depth(list(range(1, 11)), length=5, max_depth=3) >>> assert data == [1, 2, 3, 4, 5, [6, 7, 8, 9, 10]] >>> data = create_data_increasing_depth(list(range(1, 11)), length=2, max_depth=3) >>> assert data == [1, 2, [3, 4, [5, 6]]], 7, 8, [9, 10]] """ _result = _result or [] _current_depth = _current_depth or max_depth data = iter(data) try: _current_length = length while _current_length: item = next(data) _result.append(item) _current_length -= 1 except StopIteration: return _result if _current_depth - 1: tmp_res = create_data_increasing_depth( data=data, length=length, max_depth=max_depth, _current_depth=_current_depth - 1) if tmp_res: _result.append(tmp_res) if max_depth == _current_depth: tmp_res = create_data_increasing_depth( data=data, length=length, max_depth=max_depth) if tmp_res: _result += tmp_res return _result
此函数创建嵌套列表,嵌套增加。
>>> data = create_data_increasing_depth(list(range(1, 11)), length=5, max_depth=3) >>> assert data == [1, 2, 3, 4, 5, [6, 7, 8, 9, 10]] >>> data = create_data_increasing_depth(list(range(1, 11)), length=2, max_depth=3) >>> assert data == [1, 2, [3, 4, [5, 6]]], 7, 8, [9, 10]]
generate_data
实作 def generate_data() -> List[Tuple[str, Dict[str, Union[range, Num]]]]: """ Generated collections of Data by pattern {amount_item}_amount_{length}_length_{max_depth}_max_depth where: .. py:attribute:: amount_item: len of flatten elements .. py:attribute:: length: len of elements at the same level of nesting .. py:attribute:: max_depth: highest possible level of nesting """ data = [] amount_of_elements = [10 ** i for i in range(5)] data_template = '{amount_item}_amount_{length}_length_{max_depth}_max_depth'
此功能直接创建测试数据的模式。 为此,它将生成由模板创建的标题。
data_template = '{amount_item}_amount_{length}_length_{max_depth}_max_depth'
其中:
- 数量-列表中的项目总数
- length-一个嵌套级别的元素数
- max_depth-最大嵌套级别数
数据本身被传输到上述功能以生成必要的数据。 因此,如稍后将看到的那样,输出将具有以下数据(标题):
10_amount_1_length_1_max_depth 100_amount_1_length_1_max_depth 100_amount_1_length_10_max_depth 100_amount_3_length_10_max_depth 100_amount_6_length_10_max_depth 100_amount_9_length_10_max_depth 1000_amount_1_length_1_max_depth 1000_amount_1_length_10_max_depth 1000_amount_3_length_10_max_depth 1000_amount_6_length_10_max_depth 1000_amount_9_length_10_max_depth 1000_amount_1_length_100_max_depth 1000_amount_25_length_100_max_depth 1000_amount_50_length_100_max_depth 1000_amount_75_length_100_max_depth 1000_amount_100_length_100_max_depth 10000_amount_1_length_1_max_depth 10000_amount_1_length_10_max_depth 10000_amount_3_length_10_max_depth 10000_amount_6_length_10_max_depth 10000_amount_9_length_10_max_depth 10000_amount_1_length_100_max_depth 10000_amount_25_length_100_max_depth 10000_amount_50_length_100_max_depth 10000_amount_75_length_100_max_depth 10000_amount_100_length_100_max_depth 10000_amount_1_length_1000_max_depth 10000_amount_250_length_1000_max_depth 10000_amount_500_length_1000_max_depth 10000_amount_750_length_1000_max_depth 10000_amount_1000_length_1000_max_depth
第3部分。结果
要分析CPU,我使用了line_profiler
用于绘图-timeit + matplotlib
CPU Profiler
结论 $ kernprof -l funcs.py $ python -m line_profiler funcs.py.lprof Timer unit: 1e-06 s Total time: 1.7e-05 s File: funcs.py Function: outer_flatten_1 at line 11 Line # Hits Time Per Hit % Time Line Contents ============================================================== 11 @profile 12 def outer_flatten_1(array: Iterable) -> List: 13 """ 14 Based on C realization of this solution 15 More on: 16 17 https://iteration-utilities.readthedocs.io/en/latest/generated/deepflatten.html 18 19 https://github.com/MSeifert04/iteration_utilities/blob/384948b4e82e41de47fa79fb73efc56c08549b01/src/deepflatten.c 20 """ 21 2 17.0 8.5 100.0 return deepflatten(array) Total time: 3.3e-05 s File: funcs.py Function: outer_flatten_2 at line 24 Line # Hits Time Per Hit % Time Line Contents ============================================================== 24 @profile 25 def outer_flatten_2(array: Iterable) -> List: 26 """ 27 recursive algorithm, vaguely reminiscent of recursion_flatten. Based on next pattern: 28 29 .. code:: python 30 31 try: 32 tree = iter(node) 33 except TypeError: 34 yield node 35 36 more on: 37 https://more-itertools.readthedocs.io/en/stable/api.html#more_itertools.collapse 38 """ 39 2 33.0 16.5 100.0 return collapse(array) Total time: 0.105099 s File: funcs.py Function: niccolum_flatten at line 42 Line # Hits Time Per Hit % Time Line Contents ============================================================== 42 @profile 43 def niccolum_flatten(array: Iterable) -> List: 44 """ 45 Non recursive algorithm 46 Based on pop/insert elements in current list 47 """ 48 49 2 39.0 19.5 0.0 new_array = array[:] 50 2 6.0 3.0 0.0 ind = 0 51 2 2.0 1.0 0.0 while True: 52 20002 7778.0 0.4 7.4 try: 53 21010 13528.0 0.6 12.9 while isinstance(new_array[ind], list): 54 1008 1520.0 1.5 1.4 item = new_array.pop(ind) 55 21014 13423.0 0.6 12.8 for inner_item in reversed(item): 56 20006 59375.0 3.0 56.5 new_array.insert(ind, inner_item) 57 20000 9423.0 0.5 9.0 ind += 1 58 2 2.0 1.0 0.0 except IndexError: 59 2 2.0 1.0 0.0 break 60 2 1.0 0.5 0.0 return new_array Total time: 0.137481 s File: funcs.py Function: tishka_flatten at line 63 Line # Hits Time Per Hit % Time Line Contents ============================================================== 63 @profile 64 def tishka_flatten(data: Iterable) -> List: 65 """ 66 Non recursive algorithm 67 Based on append/extend elements to new list 68 69 """ 70 2 17.0 8.5 0.0 nested = True 71 1012 1044.0 1.0 0.8 while nested: 72 1010 1063.0 1.1 0.8 new = [] 73 1010 992.0 1.0 0.7 nested = False 74 112018 38090.0 0.3 27.7 for i in data: 75 111008 50247.0 0.5 36.5 if isinstance(i, list): 76 1008 1431.0 1.4 1.0 new.extend(i) 77 1008 1138.0 1.1 0.8 nested = True 78 else: 79 110000 42052.0 0.4 30.6 new.append(i) 80 1010 1406.0 1.4 1.0 data = new 81 2 1.0 0.5 0.0 return data Total time: 0.062931 s File: funcs.py Function: zart_flatten at line 84 Line # Hits Time Per Hit % Time Line Contents ============================================================== 84 @profile 85 def zart_flatten(a: Iterable) -> List: 86 """ 87 Non recursive algorithm 88 Based on pop from old and append elements to new list 89 """ 90 2 20.0 10.0 0.0 queue, out = [a], [] 91 21012 12866.0 0.6 20.4 while queue: 92 21010 16849.0 0.8 26.8 elem = queue.pop(-1) 93 21010 17768.0 0.8 28.2 if isinstance(elem, list): 94 1010 1562.0 1.5 2.5 queue.extend(elem) 95 else: 96 20000 13813.0 0.7 21.9 out.append(elem) 97 2 53.0 26.5 0.1 return out[::-1] Total time: 0.052754 s File: funcs.py Function: recursive_flatten_generator at line 100 Line # Hits Time Per Hit % Time Line Contents ============================================================== 100 @profile 101 def recursive_flatten_generator(array: Iterable) -> List: 102 """ 103 Recursive algorithm 104 Looks like recursive_flatten_iterator, but with extend/append 105 106 """ 107 1010 1569.0 1.6 3.0 lst = [] 108 22018 13565.0 0.6 25.7 for i in array: 109 21008 17060.0 0.8 32.3 if isinstance(i, list): 110 1008 6624.0 6.6 12.6 lst.extend(recursive_flatten_generator(i)) 111 else: 112 20000 13622.0 0.7 25.8 lst.append(i) 113 1010 314.0 0.3 0.6 return lst Total time: 0.054103 s File: funcs.py Function: recursive_flatten_iterator at line 116 Line # Hits Time Per Hit % Time Line Contents ============================================================== 116 @profile 117 def recursive_flatten_iterator(arr: Iterable) -> Iterator: 118 """ 119 Recursive algorithm based on iterator 120 Usual solution to this problem 121 122 """ 123 124 22018 20200.0 0.9 37.3 for i in arr: 125 21008 19363.0 0.9 35.8 if isinstance(i, list): 126 1008 6856.0 6.8 12.7 yield from recursive_flatten_iterator(i) 127 else: 128 20000 7684.0 0.4 14.2 yield i Total time: 0.056111 s File: funcs.py Function: tishka_flatten_with_stack at line 131 Line # Hits Time Per Hit % Time Line Contents ============================================================== 131 @profile 132 def tishka_flatten_with_stack(seq: Iterable) -> List: 133 """ 134 Non recursive algorithm 135 Based on zart_flatten, but build on try/except pattern 136 """ 137 2 24.0 12.0 0.0 stack = [iter(seq)] 138 2 5.0 2.5 0.0 new = [] 139 1012 357.0 0.4 0.6 while stack: 140 1010 435.0 0.4 0.8 i = stack.pop() 141 1010 328.0 0.3 0.6 try: 142 1010 330.0 0.3 0.6 while True: 143 22018 17272.0 0.8 30.8 data = next(i) 144 21008 18951.0 0.9 33.8 if isinstance(data, list): 145 1008 997.0 1.0 1.8 stack.append(i) 146 1008 1205.0 1.2 2.1 i = iter(data) 147 else: 148 20000 15413.0 0.8 27.5 new.append(data) 149 1010 425.0 0.4 0.8 except StopIteration: 150 1010 368.0 0.4 0.7 pass 151 2 1.0 0.5 0.0 return new
图表
总体结果:
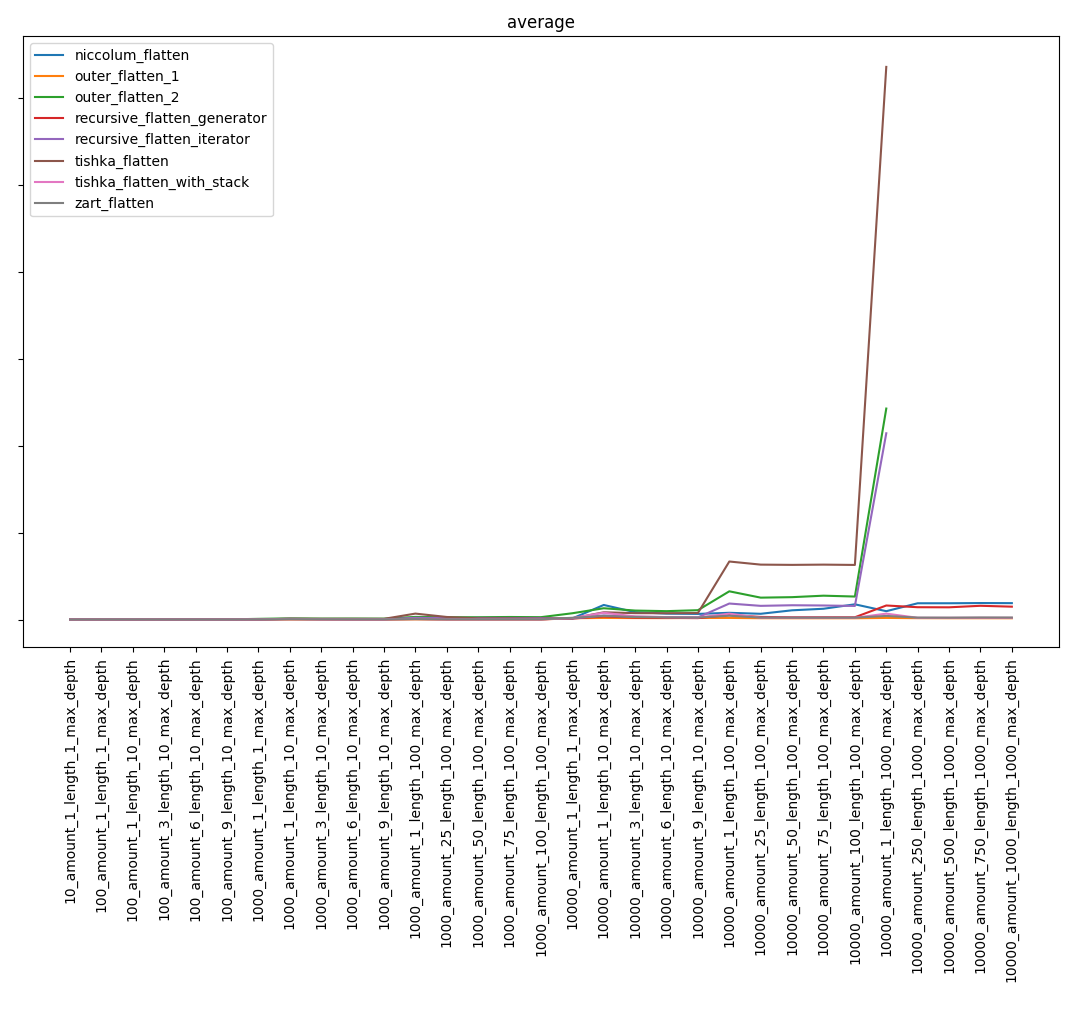
排除最慢的功能,我们得到:
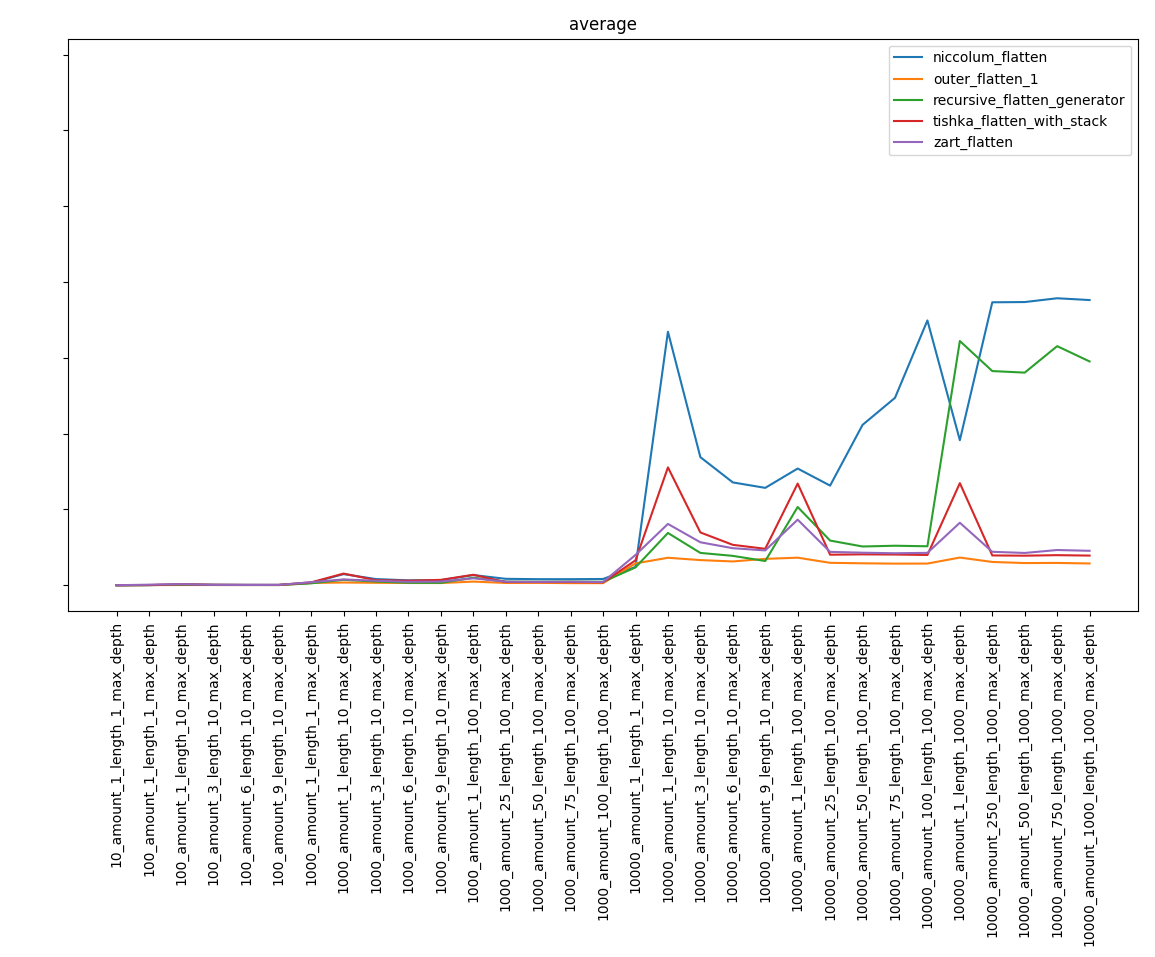
第4部分。结论
也许我会说显而易见的事情,但是,尽管算法复杂,但结果可能并不明显。 因此,复杂度最高的niccolum_flatten函数进入了最后阶段,并且距离最后一步很远。 而且事实证明recursive_flatten_generator比recursive_flatten_iterator要快得多。
总结一下,我首先要说的是,这更多的是研究,而不是编写有效列表解压缩算法的指南。 通常,您可以编写最简单的实现,而无需考虑它是否最快,因为 节省很少。
有用的链接
在这里可以找到更完整的结果。
此处包含代码的存储库
通过sphinx的文档在这里
如果发现任何错误,请写信给Niccolum电报或lastsal@mail.ru。
我很乐意接受建设性的批评。