预期“开发人员算法”课程的开始,他们准备了另一篇有趣的文章的翻译。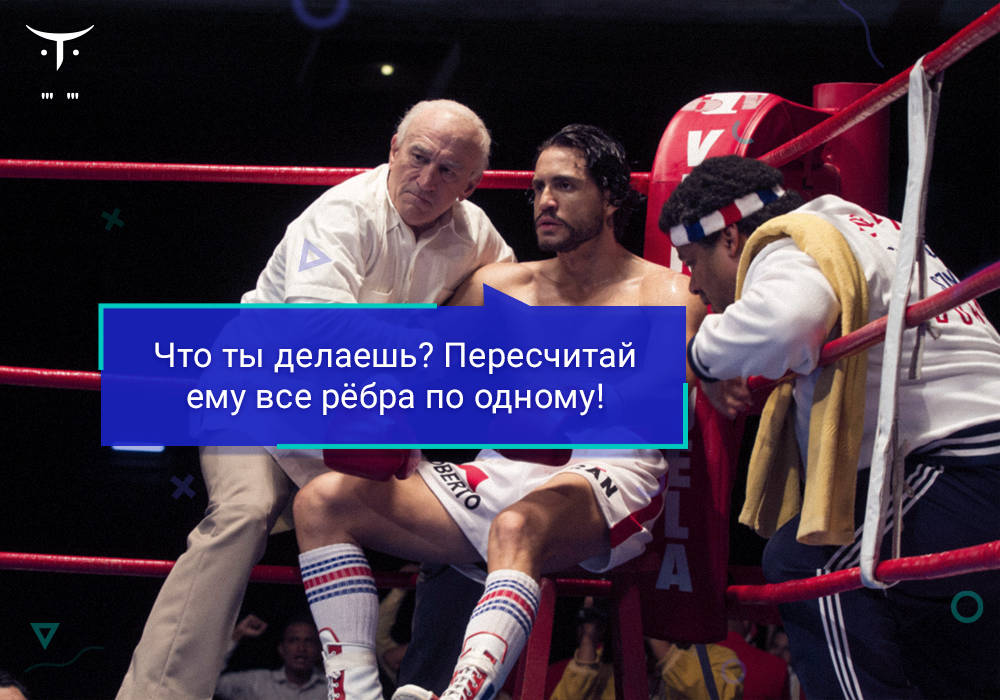
问题 :给定一个图和一个图的初始顶点src,有必要找到从src到给定图中所有顶点的最短路径。 该图可能包含负权重的边。
我们已经讨论了Dijkstra算法作为解决此问题的一种方法。 Dijkstra的算法是贪婪算法,其复杂度为O(VLogV)(使用斐波那契堆)。 但是,Dijkstra不适用于负负权重的图,而Bellman-Ford完全适用。 Bellman-Ford算法甚至比Dijkstra算法更简单,非常适合于分布式系统。 同时,它的复杂度为
O(VE) ,这比Dijkstra算法的指标还高。
建议 :在继续查看解决方案之前,请尝试
练习 。
演算法
以下是详细步骤。
输入 :图形和初始顶点
src
。
输出 :距src到所有顶点的最短距离。 如果出现负重的循环,则不会计算最短距离,将显示一条消息,指示存在这种循环。
- 在此步骤中,从初始顶点到所有其他顶点的距离被初始化为无穷大,并且到src本身的距离被假定为0
|V|
所有值都等于无穷大,但dist[src]
元素除外,其中src
是原始顶点。 - 第二步计算最短距离。 必须执行以下步骤
|V|
-1次,其中|V|
-此图中的顶点数。
- 对每个uv边缘执行以下操作:
如果dist[v] > dist[u] + uv
,则更新dist[v]
dist [v] = dist [u] + uv
- 在此步骤中,报告图表中是否存在负重量循环。 对于每个uv边缘,执行以下操作:
- 如果
dist[v] > dist[u] + uv
,则该图包含负权重的循环。
步骤3的想法是,如果图形不包含负权重的循环,则步骤2保证最短距离。 如果我们再次遍历所有边缘,并且获得任意顶点的较短路径,则这将表示存在负权重循环。
如何运作? 与其他动态编程任务一样,该算法从下至上计算最短路径。 首先,它计算最短距离,即,路径的长度不超过一条边。 然后,它计算长度不超过两个边的最短路径,依此类推。 在外循环的第i次迭代之后,将计算长度不超过
i个边的最短路径。 在任何简单路径中,最多可以有
| V | -1个边,因此外循环正好运行
| V | -1次。 这个想法是,如果我们计算不超过
i个边的最短路径,那么对所有边进行迭代就可以确保获得不超过
i +1个边的最短路径(证明很简单,您可以参考
MIT的 本次讲座或
视频讲座 )
例子
让我们在下面的图形示例中查看算法。
从这里拍摄的图像。
令初始顶点为0。除与
src
本身的距离外,所有距离均为无穷大。 图中的顶点总数为5,因此所有边都需要移动4次。

按以下顺序计算肋骨:(B,E),(D,B),(B,D),(A,B),(A,C),(D,C),(B,C),( E,D)。 当沿肋骨的通道首次完成时,我们得到以下距离。 第一行显示初始距离,第二行显示在处理边缘(B,E),(D,B),(B,D)和(A,B)时的距离。 第三行显示处理距离(A,C)。 第四行显示处理(D,C),(B,C)和(E,D)时发生的情况。

第一次迭代可确保所有最短路径不超过1条边的路径。 当所有边缘的第二次通过完成时,我们得到以下距离(最后一行显示最终值)。

第二次迭代确保所有最短路径的长度最多为2个边。 该算法再沿所有边缘运行2次。 在第二次迭代后将距离最小化,因此第三次和第四次迭代不会更新距离值。
实现方式:
输出值:
注意事项:- 在各种图形应用程序中都可以找到负权重。 例如,除了增加一条路径的成本,我们还可以通过遵循一条特定的路径而受益。
- Bellman-Ford算法在分布式系统上效果更好(比Dijkstra算法更好)。 与Dijkstra不同,我们需要找到所有顶点的最小值,在Bellman Ford中,边缘一次被视为一个。
练习:- 标准的Bellman-Ford算法仅在不具有负权重循环的情况下才报告最短路径。 对其进行修改,即使存在这样的循环,也可以报告最短的路径。
- 我们可以使用Dijkstra算法在权重为负的图中找到最短路径吗? 有这样一个想法:计算最小权重值,对所有权重添加一个正值(等于最小权重值的绝对值),并对修改后的图运行Dijkstra算法。 这样的算法会起作用吗?
Bellman-Ford算法的简单实现资料来源:www.youtube.com/watch?v=Ttezuzs39nkzh.wikipedia.org/wiki/Bellman%E2%80%93Ford_algorithmwww.cs.arizona.edu/classes/cs445/spring07/ShortestPath2.prn.pdf